The future of AI in biologics drug discovery
The future of AI in biologics drug discovery
By Sam DeLuca, Ph.D. With Steven Lewis, Ph.D. and Lucas Nivon, Ph.D.
June 2023
In our previous article in March 2023, we discussed our experience benchmarking publicly available AI protein design tools and exploring their real world strengths and weaknesses. Here, we want to be more speculative and lay out a vision for how we think these AI methods will impact the future development of protein engineering and the resulting clinically meaningful biologics.
AI Structure Prediction as a rapid hypothesis test
While AlphaFold2, OpenFold, and ProteinMPNN have led headlines with their remarkable effectiveness at making accurate protein structure and sequence predictions, an underappreciated impact of these tools is their speed. These tools have reduced the amount of computational time required for comparative modeling (protein structure prediction via traditional homology and physics methods) and protein design tasks. As a rough example, a comparative modeling job which would have taken thousands of hours of CPU time using Rosetta in 2010 and hundreds of hours of CPU time in 2018 now takes only 1-2 hours of CPU/GPU time using AlphaFold2 or OpenFold with a reasonably powerful GPU like a Tesla T4. The most obvious impact of this drastic reduction of compute time is that researchers can now perform modeling experiments on a vast scale, such as the AlphaFold Protein Structure Database.
A somewhat less obvious impact of the reduction of compute time is the reduction in level of effort, cost, and impact of homology modeling of a single protein. Although academic use of homology modeling via free online servers like Robetta has been straightforward for 20 years, license and data security requirements have kept the tool out of the hands of industry. More recently, Cyrus Bench alleviated this. Still, running homology modeling yourself on local secure resources was a major effort, requiring scientists with specialized training and substantial analysis and post-processing effort. This level of effort limited the use of comparative modeling in industrial settings, where access to large scale compute hardware is more expensive relative to academic research facilities.
Current ML tools have effectively eliminated this limitation. When comparative modeling is trivial both from a technical and a cost standpoint, it becomes an obvious first step in any molecular modeling project. AlphaFold2 and OpenFold do not always produce useful output, and their output needs to be carefully scrutinized before use, but for native proteins the vast majority of the time they result in a model which can provide at least some useful structural insights, and frequently produce models that are directly useful in protein engineering. The ability to produce usable models cheaply and rapidly also opens the door to novel uses of comparative modeling, such as validation of de novo protein designs, as we have seen now extensively from the Baker Lab at the University of Washington.
We still need physics
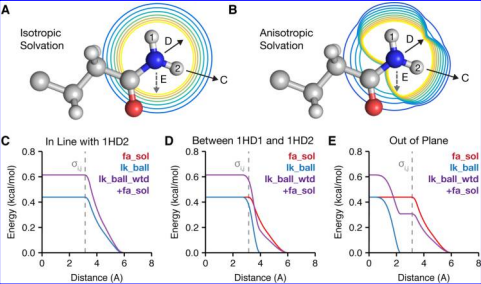
Despite the power of molecular modeling techniques for rapidly predicting sequences and structures, they remain inferior to statistical physics based methods at ranking models by stability or activity and differentiating between models of very similar sequence. For this, it is still important to use a more traditional physics or knowledge based scoring function (such as the Rosetta hybrid statistical/physical energy function or a “pure physics” molecular mechanics force field, or a combination thereof).
Additionally, machine learning methods are generally incapable of modeling outside of the domain of their training data, and thus for the foreseeable future it will be necessary to use more traditional methods to model novel small molecule and Non-Canonical Amino Acid (NCAA) chemistry. Small molecule and NCAA modeling are therefore of high priority for the OpenFold Consortium which Cyrus co-founded with Genentech/Prescient, Outpace, and Arzeda.
We still need experimental validation in the biochemistry lab and in animals
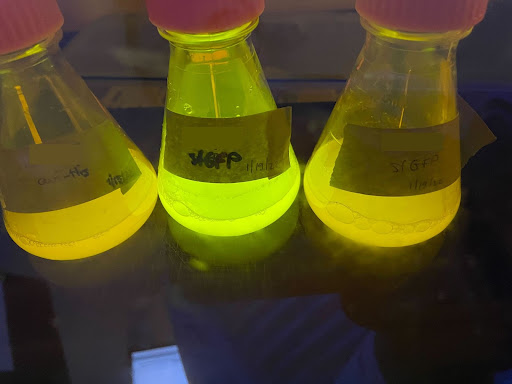
A designed protein operates in a complex biological environment, and in most medically relevant cases that environment is only partially understood. For this reason, experimental validation of designs will always be a necessary component of ML driven protein design, regardless of the accuracy of the ML model, because those ML models are trained on examples of purified proteins in artificial buffers, not on the behavior of proteins inside a cell, let alone a protein inside a living mouse or human.
In Cyrus’ experience, to make the best use of computational protein design tools it is necessary to have an efficient, closely collaborative loop between computational design and experimental validation. If well executed, this loop creates a virtuous cycle in which the results of the experimental validation provide data and insights which are used to fine-tune future rounds of computational design, and the computational design provides insights into the structure and chemistry of the designed proteins that can improve experimental methods. Along the way, the core algorithms are expanded and become more capable for future projects.
Establishing the “primitives” of protein engineering
Given these new tools, it’s worth taking a step back and considering what a comprehensive tool for protein engineering might look like in the near future. To begin with, let us compare the basic user interface of Cyrus Bench, the GUI protein engineering tool which Cyrus developed before any of the current ML tools existed, and Autodesk, an industry standard CAD tool:
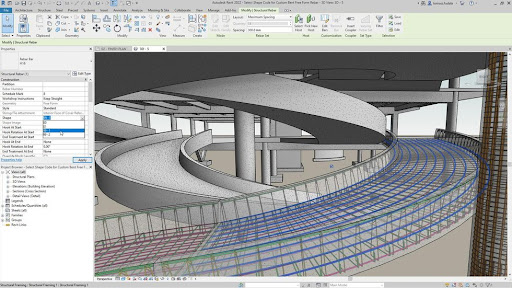
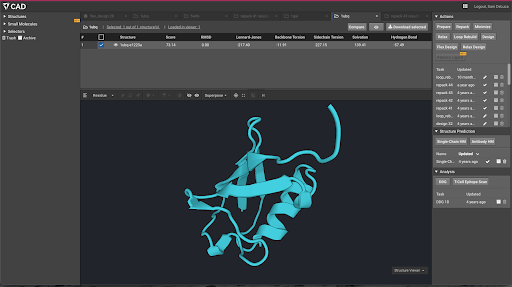
The basic UI concept between these two applications is intentionally similar, with a model (a building for Autodesk, a protein structure for Bench) being designed at the center of the user interface, and a palette of tools to manipulate that structure (e.g. extrusion of a 3D shape for Autodesk, loop rebuilding for a protein in Bench.
Inspection of the actual tools available, however, indicates a major difference between the user interactivity in the two tools – Autodesk is fast and responsive, Bench is mostly running slow calculations on its huge cloud back end. The tools available in Autodesk (and in most other mature engineering, art, and design tools) are relatively simple primitives like “extrude”, “rotate”, etc. The user is given a palette of fast running primitives to manipulate their model, and a larger set of analysis and measurement tools to analyze and validate that model. The vast majority of the tools in Bench take minutes to hours to run, even with tens to hundreds of CPUs calculating on the backend, and therefore most user interaction is asynchronous.
(Note: The authors of this blog post is a structural biologist and software engineer, not a mechanical engineer and has only a superficial understanding of actual CAD software).
The advantage of simpler individual tools is that they allow the user more flexibility and interactive reasoning in their usage of the tool. From a user experience perspective, these tools need to run very quickly in order for them to be usable. Historically, molecular modeling methods have been far too long-running and computationally expensive for this sort of simple tool user interface to be feasible. However, the dramatic reduction in the amount of time necessary to perform a single modeling action over the past several years opens the possibility of making far more interactive and fluid protein modeling tools than were previously possible.
In a hypothetical purely AI-driven future for protein design, then,fasst-running tools could be exposed to domain-expert users directly. Assuming those tools have been vetted for useful outputs, as we discussed in our last blog post, those users will be able to rapidly iterate on a protein design while having a reasonable expectation that the outputs will behave as desired in the lab.
You get what you train for: Data will drive progress
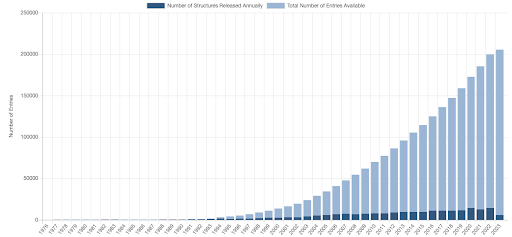
As of this writing in mid 2023, some of the tools for protein design remain in the domain of physics/statistics, while some are now fully AI/deep learning tools that have replaced older tools. Roughly speaking this process is 50% complete. Structure prediction and design of full de novo proteins and heavily-redesigned proteins (changing more than 50% of the residues in a protein) are now all tools in the realm of AI. Redesign of natural proteins is still mostly not AI driven, especially if a crystal structure exists of the starting protein – this includes the design for point mutations to increase stability or affinity, design of solubility, or design of glycosylation.
Over time as datasets grow, and new ways of collecting large-scale data are developed, we expect the applicable domain of AI methods in molecular modeling to grow. This seems to be happening with protein stability. Work such as Gabe Rocklin’s at the University of Chicago in “mega-scale protein design” is paving the way to improved AI driven protein stability models. We see this area as a very fruitful collaborative area between academia and industry, where open data sources can lead to massively improved open models, including through the AI consortium we cofounded, OpenFold. Other datasets in protein/ligand binding or antibody structure could also lead to new models for small molecule drug development and biologics development, respectively.
All of these AI models are driven by the large scale, accurate, physiologically-relevant data that underlie them. David Baker and Minkyung Baek theorized about some of these future directions in Nature in early 2022. In areas where we can collect millions of high-quality, diverse data points, we expect AI models to eventually replace older models. In other areas where it may be difficult or impossible to generate such data, we may continue to use physical models, hybrid statistical/physical models, or even quantum mechanical models.
The generation of the underlying datasets, whether across large sets of proteins or just for one family of proteins, will drive further general advances and help optimize specific proteins as drugs. Cyrus’ principle focus for dataset generation is deep mutational scanning for individual proteins of therapeutic interest, so we can create superior biologics that are optimized across sequence space for maximal efficacy, high specificity, and few liabilities that could hinder clinical development.
Going to the clinic
The measure of our field’s work in AI-driven biologics discovery will be in it’s clinical impact, not in the diversity of our models or our ability to generate data. Here we’ve outlined the huge changes that AI structure prediction and de novo design have already made, the continuing role of statistics and physics in biologics modeling, the necessity of iterations between algorithm and laboratory, the changes resulting from faster AI methods, and where our core AI models will be developing next. We are excited to move superior biologics into the clinic at Cyrus, and for the progress that the next 5 years will bring across the BioPharma industry as a variety of firms bring new AI designed drugs to clinical trials and into patient’s lives.